Revolutionizing Reality: The Surge of AI-Powered Generative Technologies
In the dynamic world of tech, few innovations have surged as powerfully and swiftly as AI-powered generative technologies. These systems, particularly those based on advanced deep learning architectures, are transforming how content is created, from text and images to music and even complex 3D environments. Behind this surge lies a sophisticated mesh of algorithms known as generative adversarial networks (GANs), transformers, and diffusion models, which together push the boundaries of what machines can imagine and produce.
At the heart of generative AI’s rise is its ability to mimic human creativity—a task once believed to be exclusively human. These technologies do not simply replicate data; they analyze vast datasets, learn underlying patterns, and generate novel outputs with striking originality. OpenAI’s GPT series, including GPT-4, has set new benchmarks in natural language generation, enabling not only coherent writing but also nuanced conversations, problem-solving, and creative storytelling. Meanwhile, tools like DALL·E and Stable Diffusion have ushered in a new era of image generation, allowing anyone to conjure intricate visuals from simple textual prompts.
Applications Across Industries: From Art to Automation
The implications of AI generative tech ripple across numerous industries, redefining workflows, enhancing productivity, and spawning innovative business models. In entertainment, filmmakers and game developers exploit these tools to create complex narratives and immersive environments quickly and cost-effectively, reducing reliance on extensive human labor without sacrificing quality. Video game studios now employ AI to generate non-player characters with dynamic dialogues and behaviors, producing more realistic and personalized gaming experiences.
Advertising and marketing have also embraced generative AI to craft targeted content tailored to consumer preferences. Automated copywriting and personalized visual ads enable brands to scale campaigns with unprecedented speed and precision. Beyond creative fields, AI-generated synthetic data proves invaluable in sectors like healthcare and finance, where real-world data privacy and scarcity limit traditional training approaches. Synthetic datasets help train models for diagnostic imaging or fraud detection while preserving patient and client confidentiality.
In robotics, generative models contribute to designing novel parts and optimizing control systems. AI-driven simulations test thousands of robotic configurations virtually before production, accelerating innovation cycles. In manufacturing, AI-generated schematics streamline prototyping, underscoring generative models’ vital role in physical product development.
Societal Impact: Empowerment and New Challenges
The democratization of content creation offered by generative AI profoundly impacts society, empowering individuals and small enterprises to produce professional-grade work without extensive resources. Writers, artists, and musicians harness AI as a collaborator, unlocking new creative pathways. Educational tools powered by generative models provide personalized tutoring and curriculum generation, fostering inclusive learning environments.
However, this technological leap also surfaces critical ethical and societal challenges. The ease with which AI can fabricate hyper-realistic images, videos, and audio has intensified concerns about misinformation, deepfakes, and the erosion of trust in digital media. As AI systems become more adept at mimicking human outputs, distinguishing authentic content from synthetic artifacts grows increasingly difficult.
Furthermore, the massive resource consumption required to train and deploy large generative models raises questions about sustainability within the tech industry. Carbon footprints associated with extensive computing tasks necessitate innovative strategies to balance progress with environmental responsibility.
Ethical Considerations: Towards Responsible Innovation
Navigating the ethical landscape of generative AI calls for rigorous frameworks that address bias, accountability, and transparency. These models inherit biases present in their training datasets, potentially propagating stereotypes or misinformation. Developers and researchers collaborate to improve data diversity, implement fairness constraints, and provide interpretability tools that illuminate decision-making processes.
Intellectual property rights represent another complex area as AI-generated content challenges existing copyright laws. Who owns an AI-generated artwork or text—the user, developer, or the AI itself? Legal scholars and policymakers engage in ongoing debates to reshape regulations that reflect these contemporary realities.
Leading tech organizations actively contribute to responsible AI development. OpenAI emphasizes ethical guidelines and broad societal benefit, while DeepMind and NVIDIA work towards fairness and sustainability in model design. Partnerships between academia, industry, and governments strive to establish standards ensuring generative AI serves humanity’s best interests.
Driving Innovation: Key Players and Breakthroughs
The explosive growth of generative AI is anchored by pivotal contributions from tech giants and innovative startups alike. OpenAI’s release of GPT models has galvanized the field, setting new performance and accessibility standards. Google’s DeepMind and its innovations with transformer architectures have propelled natural language processing to new heights. Meanwhile, companies like Stability AI and Midjourney offer democratized access to image generation tools, catalyzing grassroots creativity.
Academic institutions fuel theoretical advancements, exploring novel architectures and refining training methodologies. Research published in venues like NeurIPS and ICML continually pushes the envelope, prompting industry application and commercialization.
Hardware advancements are equally vital; specialized AI accelerators developed by NVIDIA, AMD, and Google’s TPU team enable the computational heft needed for generative models. These collaborations between hardware and software innovators constitute the backbone of current and future breakthroughs.
The Road Ahead: Toward Synergistic Human-Machine Creativity
As generative AI technologies mature, the future landscape will likely feature deeper integration with human creative processes, fostering symbiotic relationships rather than simple automation. Visionaries forecast AI tools evolving to anticipate human intent, creatively augmenting ideas and workflows with increasing subtlety and contextual awareness.
Emerging research into multimodal generative systems promises unified models that seamlessly combine text, image, audio, and even tactile information, revolutionizing interfaces and experiences. Imagine virtual environments generated in real-time that adapt to a user’s narrative choices, or AI-powered design assistants that prototype architectural structures blending aesthetic and engineering criteria flawlessly.
Moreover, continued emphasis on ethical stewardship and equitable access will shape how technology influences societal trajectories. Interdisciplinary collaboration will be crucial to harness generative AI’s immense potential while mitigating its risks.
The surge of AI-powered generative tech marks a monumental chapter in the evolution of technology — one that challenges traditional boundaries of creativity and intelligence. By exploring its real-world applications, acknowledging ethical complexities, and spotlighting key innovators, we gain a comprehensive understanding of this transformative force poised to redefine the fabric of human expression and productivity in the digital era.
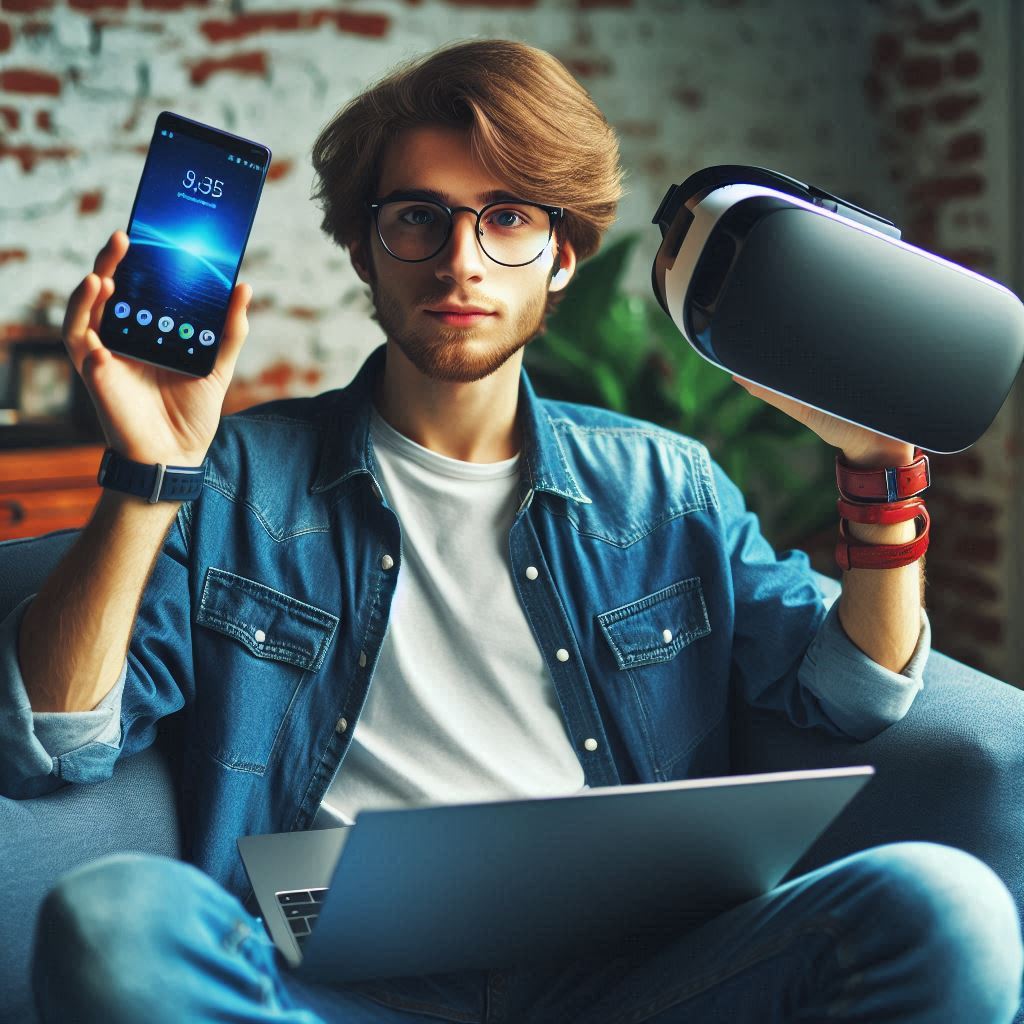